Can Artificial Intelligence Outperform Humans?
Before a cancer patient undergoes radiation or surgery, it is imperative to identify the tumor’s borders to determine its exact location and avoid damaging surrounding areas. This is especially difficult for brain tumors, which are very diffuse and often extend out into healthy tissue.
Even using multiple MRI scans, it’s time consuming and challenging for top experts in the field to nail down the precise borders of brain tumors. In most cases, scientists use a consensus from multiple radiologists to approximate the tumor border in large research studies.
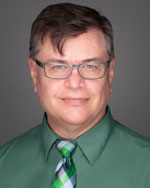
J. Ross Mitchell, PhD, Artificial Intelligence Officer
“The issue with that is, first of all, we don’t really know that’s the ‘truth.’ Nobody really knows where the border is,” said Dr. J. Ross Mitchell, Artificial Intelligence officer at Moffitt Cancer Center. “Second of all, it’s very time consuming and expensive to get highly-trained expert neuro-radiologists together to come to a consensus over hundreds of patient cases.”
Artificial Intelligence (AI) has become a proven tool to help with outlining brain structures and lesions, a process called segmentation. In a matter of seconds, a deep learning network is able to analyze MRIs to locate and segment brain tumors.
However, Moffitt researchers wanted to take it one step farther. While previous studies used at least four high-quality scans per patient to train the deep learning network, the Moffitt team used two clinical-grade scans from patients acquired any time over the last 30 years. This type of data would be more commonly seen in busy clinics around the country.
“Our data set is very diverse in terms of its age, the span of time, the number of centers the data is from and the manufacturers of the scanners, since the equipment itself changed dramatically over three decades,” said Mitchell.
While training the deep learning network this way was more challenging, the study results showed it was on par with the state-of-the-art networks trained at large centers with very specific data. Even though the results were good, the research team wanted to look at the errors to determine where the deep learning network was making mistakes.
Mitchell created a system that allowed 20 experts to do a blind side-by-side comparison of borders created by humans versus AI. They scored the quality of each outline on a scale of zero to 10. In the end, the experts ranked AI higher on average than the technicians.
“This is the first time a side-by-side comparison, an independent adjudication by experts, has been done,” said Mitchell. “And that’s important because it’s a new standard for evaluating how these things perform.”
Prior to this study, it was the common belief that a machine learning model is only as good as the data used to train it. However, that isn’t necessarily true. “This is the first study that shows AI can learn to be better than the human who trained it at outlining structures in medical images,” said Mitchell. “AI can see through mistakes made by humans and learn to ignore them.”
The study has major implications in medicine moving forward. It shows that health care institutions can build a robust deep learning network using ordinary data and easily tune the application to fit individual needs.
Not only can AI become a valuable tool in the clinic to help segment brain tumors, but Mitchell also would like to use this study to build a program he could provide to other hospitals. It could also be used in the future to segment other cancer types.
“If I am going to build something to let’s say segment prostate cancer, I may not need to have the best experts in the world, or even the top doctors in my hospital, to gather a consensus about where the tumor is,” said Mitchell. “I might be able to get 10 technicians to do it and it would take much less time and cost much less. And the technician’s results could be used to train an AI tool that is as good or even better than the technicians themselves.”