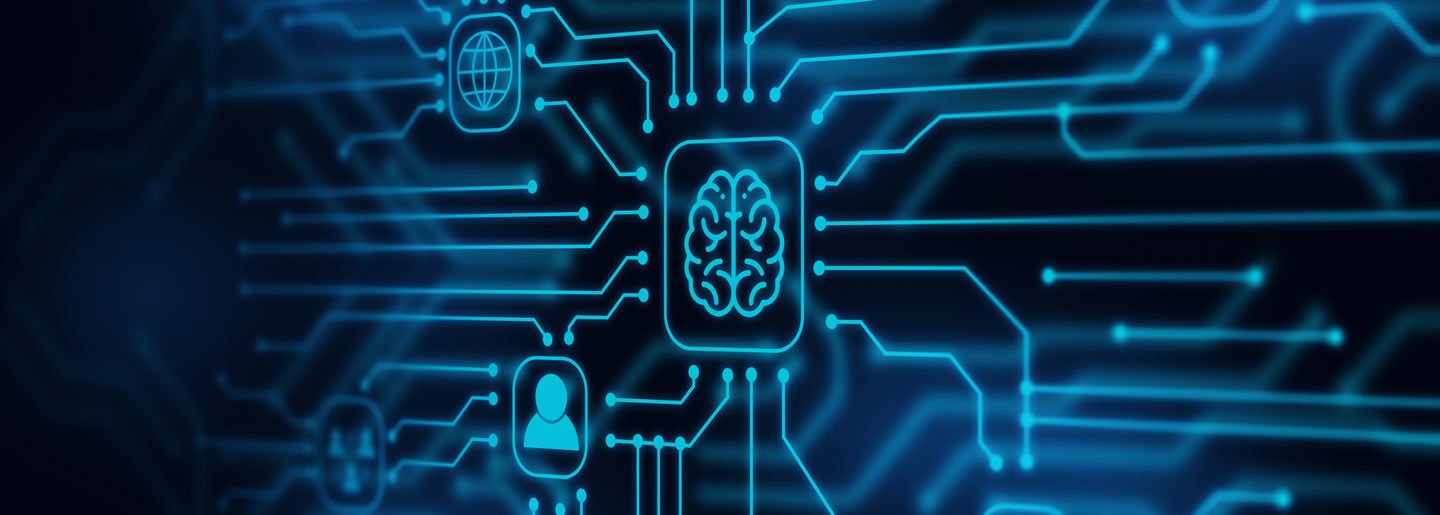
Why We Built the First Machine Learning Department in Oncology
Imagine a future where cancer patients are able to receive the treatment they precisely need and not the treatment that is prescribed to all.
Personalized medicine has advanced over recent decades but is still limited by the ability to navigate the complex landscape of patient information and tumor-specific parameters that need to be incorporated into optimizing treatment decisions.
Eliminating cancer mortality will only be possible through cancer prevention and treatment tailored not only to molecular aspects of a (pre-)malignancy, but also to the microenvironment, genetics and physiology of the patient, lifestyle factors, environmental exposures, social determinants of the patient’s health and the dynamic changes of these parameters over time.
Such a future is possible at the intersection of recent advances in computer engineering, data science and cancer research.
Conventional approaches to unraveling the complexity of tumor biology have historically focused on simple factors operating within a single scale. Advances in imaging and biotechnology have led to an exponential growth in patient-specific information, especially in oncology, ushering in a new era of personalized treatment and precision medicine for cancer patients.
However, making sense of such large and heterogenous pools of complex multi-scale multi-omics data (radiomics, genomics, proteomics, transcriptomics, etc.) requires new sets of tools beyond the traditional bioinformatic data analytic pipelines.
Emerging fields such as “radio-genomics” seek to bridge these scales, but none simultaneously embrace the entire spectrum of tumor spatiality, its microenvironment, the physiology of the patient and environmental factors that work in concert to cause cancer and drive its evolution. Furthermore, dynamic changes over time are just starting to be modeled and understood, and usually, this is within a single scale.
Nobel Laureate Dr. Philip Sharp has described the new notion of Convergence as “the interdisciplinary engagement of physics, computation, big data and engineering into the biomedical sciences… the integration of knowledge from recent technology advances—such as sequencing information from genomes and single cells, imaging modalities that can penetrate deep into the body, and utilization medical records that now span decades—… to better understand and treat cancer.”
Indeed, the evolution of cancer from a normal cell to a pre-malignant lesion to a tumor that ultimately may or may not respond to treatment and that may eventually develop resistance to therapy is determined by a complex number of factors operating across multiple scales that can be measured using various technologies (Figure 1).
The convergence approach to cancer research requires data obtained across scales at multiple time points in large populations of individuals. In parallel, advances in artificial intelligence (AI) are required to analyze and interpret these complex multi-scale data in ways human beings cannot.
Therefore, the resurgence of AI and its machine learning (ML) algorithms, driven by advances in computing hardware and software, has created tremendous excitement for AI/ML prospects in cancer research as well as for the translation of a greater understanding of the complexities of cancer biology into novel cancer prevention, early detection and treatments delivered through informed clinical trials and routine oncology practice, not only in academic centers but also in the catchment area communities, thus closing the health disparity gap across the cancer continuum.
It is recognized that AI/ML are increasingly becoming an essential part of our modern daily life, from consumer electronics to self-driving cars, and the question to pose is: Shouldn’t AI/ML be part of the solution to many of our lingering healthcare issues, especially in oncology?
If deployed properly, AI/ML can inherently realize in the true sense, the essence of convergence for personalized medicine and accelerated drug discovery in cancer and reap their promised benefits. For instance, cancer care delivery and treatment could be personalized to patient preferences—similar to an online shopping experience—where personalized medicine could be advanced through a better understanding of an individual’s heterogeneity across genetic, physiological, and psychosocial scales.
AI/ML have been hailed as the driving force behind the new fourth industrial revolution, reshaping the development of new technologies and guiding digital innovation. The excitement about Al/ML in oncology specifically was motivated by the ability of these digital technologies to automate laborious routine tasks, improve efficiencies, reduce costs, and enhance decision-making support of complex oncology processes, from treatment management to prediction of outcomes and adapting prescriptions over the course of treatment.
However, the anticipated implementation of transformative AI/ML in oncology has generally been limited in scope and sometimes stagnant, despite the known potentials and stated promises. Technical challenges and ethical issues have impeded broadening AI/ML from its current perceived role as a futuristic data analytic tool to the driver of more accurate cancer diagnosis, and safer and better treatments for cancer patients.
These challenges include limited sources of large and usable data, computing infrastructures, training bias, prediction uncertainties, generalizability to unseen data, and interpretability of AI/ML models.
For instance, in the case of electronic medical record (EMR) data, the missingness of data elements may itself introduce bias, where patients with complete records are more likely to be followed closely and have better outcomes. Moreover, patterns in clinical documentation may vary across disciplines and institutions, for example, in an academic medical hospital (AMH) versus a community-based practice setting, where AI/ML algorithms trained at an AMH may not be applicable to a community-based practice. Generalizability must also be considered when applying AI/ML algorithms across diverse patient populations characterized by a range of biological and sociodemographic differences.
To effectively leverage AI/ML as a methodology that both unravels the complexities of cancer biology and drives advances in highly effective, efficient and personalized cancer prevention, detection and treatment, AI/ML experts must be equipped with access to large volumes of multi-scale patient data and high-performance computing resources, and be properly positioned within a multidisciplinary and highly collaborative environment that includes other quantitative, basic, population, clinical and translational scientists, with direct connections to the oncology clinic and access to information about individuals at risk of cancer in the broader community and catchment area.
As such, a Department of Machine Learning was established as part of the Quantitative Sciences Division at Moffitt Cancer Center.
The Department of Machine Learning at Moffitt Cancer Center
Prior to 2020, the Division of Quantitative Sciences (QS) at Moffitt consisted of the Department of Biostatistics and Bioinformatics (Bio2) and the Department of Integrated Mathematical Oncology (IMO), chaired by Drs. Brooke Fridley and Alexander Anderson, respectively.
Thus, the newly founded Department of Machine Learning (ML) bridges the data-driven approaches of Bio2 with the mechanistic modeling strategies of IMO. The QS departments collectively house 27 faculty and more than 50 staff members, serving as an academic home for quantitative scientists to collaborate with each other and drive the development of innovative digital and quantitative methods that enrich research across all five CCSG Programs of Moffitt (Immuno-Oncology, Cancer Epidemiology, Molecular Medicine, Cancer Biology & Evolution, and Health Outcomes and Behavior).
QS faculty engage in a wide range of research with collaborators across Moffitt, including novel therapeutics and drug discovery, prognostic and predictive biomarker development, chemoprevention, high throughput genomics, proteomics, and metabolomics, next-generation sequencing, epidemiological studies, comparative effectiveness research, patient-reported outcomes, and innovative clinical trials such as adaptive therapies. Within all of these areas, AI/ML is poised to accelerate progress and/or drive new discoveries.
The ML Department complements and further cultivates the rich data infrastructure at Moffitt with AI/ML focused capabilities, as depicted in Figure 2, which highlights the congruence of AI/ML expertise with the institutional data and informatics ecosystem.
In 2010, Moffitt invested in an enterprise-wide data warehouse, coined the Health and Informatics (HRI) Platform, which integrates an extensive breadth of information captured for Moffitt patients across a variety of source systems, including the electronic health record (EHR), cancer registry, patient-reported data, billing, scheduling and research protocol consent. Coupled with Moffitt’s institution-wide biobanking protocol, Total Cancer Care, HRI also included information on biospecimen availability and research-grade molecular data.
In parallel with the implementation of HRI, Moffitt created a leadership position dedicated to data acquisition, architecture, governance, quality and access, now referred to as the chief data officer (CDO), and a data concierge service designed to provide patient data to investigators, now known as the Collaborative Data Services Core (CDSC).
Throughout the last decade, the CDO’s teams, in collaboration with Moffitt’s Information Technology departments, CDSC and the Biostatistics and Bioinformatics Shared Resource, have continued to evolve HRI, most recently incorporating an ‘omics data model that aligns sequencing results across clinical and research grade platforms. The next generation of HRI, the Moffitt Cancer Analytics Platform, or MCAP, is currently being implemented in the cloud, and is designed to provide state-of-the-art analytics tools to Moffitt faculty and enable access to enterprise-scale unstructured data, including text and images from the EHR.
Recognizing the critical role for AI/ML to both augment curated data available through MCAP and to accelerate its use for discovery, the CDO, Dr. Dana Rollison, who also serves as the associate center director of data science and division chief of quantitative science, set a course for creating the ML Department and launched a search for a pioneering leader of the first-of-its-kind Department of ML.
Dr. Issam El Naqa was recruited to chair the new ML Department. As a computer scientist and a medical physicist, Dr. El Naqa was suitable to not only expand Moffitt’s data science expertise in the area of AI/ML, but also to set its vision for the scientific research portfolio of the department, through the lens of clinical translation.
The department establishes pioneering areas of research excellence that embody the notion of multi-scale convergence of patient data and multi-disciplinary expertise with focused applications on outcomes and decision-making research, ML-aided imaging and digital pathology, ML-guided translational oncology (drug discovery and repurposing), to name a few.
The department is focused on five strategic priorities:
- Integration of ML into the fabric of cancer research and clinical care. This is currently being pursued by developing a robust and secure AI/ML infrastructure on-site and in the cloud, to leverage the multi-scale Moffitt’s enterprise-wide data warehouse, MCAP.
- Establishing translational ML programs in priority areas of cancer research (imaging and digital pathology, information retrieval and data integration, outcomes and behavioral sciences, molecular and computational biology). Many of these areas provide low hanging fruits where AI/ML can deliver transformative changes to bring convergence into cancer research and care.
- Developing basic ML research in pertinent areas to oncology (visual analytics and explainable AI/ML, automated ML, and information theory). Advances in these areas will help customize AI/ML for cancer research purposes and improve its adoption.
- Developing team science initiatives of ML experts, clinicians and basic research cancer scientists to tackle research problems with ML. Here it is important to build multidisciplinary digital innovation teams that identify current clinical and basic science problems and that leverage AI/ML tools to effectively resolve them.
- The ML department is founded with the vision of developing future leaders in the emerging field of ML oncology specifically, and across the spectrum of biomedicine. Notably, there is a pronounced lack of diversity in the data science field overall, thus, educational AI/ML initiatives will seek to strategically attract and cultivate trainees of diverse backgrounds in academic and non-academic settings, who do not necessarily have formal training in quantitative sciences, but express the interest and motivation to leverage these tools in their research and/or their practice. Thus, building a training/residency program in ML oncology with industrial and academic partners is a major endeavor of the new ML department that will cultivate the future and diverse generation of scientists in this rapidly evolving area to serve our societal needs.
Incorporating AI/ML discoveries into cancer control and care delivery
The underlying motivation for the aforementioned strategic priorities and activities is to successfully realize the notion of convergence by leveraging existing resources, bringing together multi-disciplinary expertise, identifying new impactful areas in which to tackle critical cancer-related questions, improving performance using ML through team science, and steadily growing AI/ML research across the cancer continuum.
Moreover, it is expected that synergistic relationships between the ML Department and Moffitt’s enterprise-wide digital innovation team led by Dr. Edmondo Robinson will facilitate translation of ML approaches into clinical care while identifying emerging clinical needs that can be prime targets or ideas for research proposals pursued by the ML Department faculty.
Thus, the current priorities are likely to adapt over time as ML becomes increasingly a more integral part of the cancer center’s research program, led by Dr. John Cleveland, and its clinical care program, led by Dr. Douglas Letson. This is alongside the emergence of new ML applications in oncology to optimize its safe development and improve its impact on patients’ outcomes and the healthcare system as envisioned by oncology thought leaders like Dr. Patrick Hwu, the CEO and president of Moffitt, and others in the community.
We firmly believe that ML will make cancer care more accessible, efficient and cost-effective, driving the new digital revolution in the fight against cancer.
To recapitulate in the eternal words of renowned physicist and cosmologist Sir Stephen Hawking, “Perhaps we should all stop for a moment and focus not only on making our AI[/ML] better and more successful but also on the benefit of humanity.”
Originally Published in Cancer Letter February 19, 2021